Overview
Welcome to the first International Competition on Exploring Dense Optical Flow for Gait Recognition. The competition will be in conjunction with IJCB 2025.
In this competition, we invite participants to innovate in gait recognition by utilizing the newly curated OUMVLP-OF dataset, which integrates dense optical flow maps with multi-view gait data. The competition includes two tracks: (i) gait identification, focused on recognizing individuals from their unique gait patterns, and (ii) gait verification, aimed at confirming whether two gait sequences are from the same person. Using the provided optical flow dataset, the competition challenges participants to develop cutting-edge solutions with potential applications in biometric security and healthcare.
Important Dates
The timeline for the competition is as follows.
- Competition Launch: 5 March, 2025
- Registration Deadline: 30 March, 2025
- Submission Deadline: 30 May, 2025
- Results Announcement: 10 June, 2025
How to Join the Competition?
The competition is open to everyone; however, members of the organizing teams are not eligible to participate.
To register and join the competition, please click Codabench OUMVLP-OF 2025.
IMPORTANT: Please register using your institutional email. Registrations with gmail.com or qq.com email addresses will not be approved. As well, the Team Leader must sign the license aggreement. If you are student, your supervisor must sign.
Data sharing Policy: : When releasing the data for the competition, participants will be required to agree to use it for academic use only. The dataset will be provided exclusively to registered participating teams and is strictly limited to use for the challenge.
If you have any questions or need further assistance, please don’t hesitate to reach out to oumvlpof.ch@gmail.com
Dataset
The competition will be based on the OUMVLP-OF (Optical Flow) dataset, which includes dense optical flow maps derived from the OUMVLP dataset as shown in Fig 1.
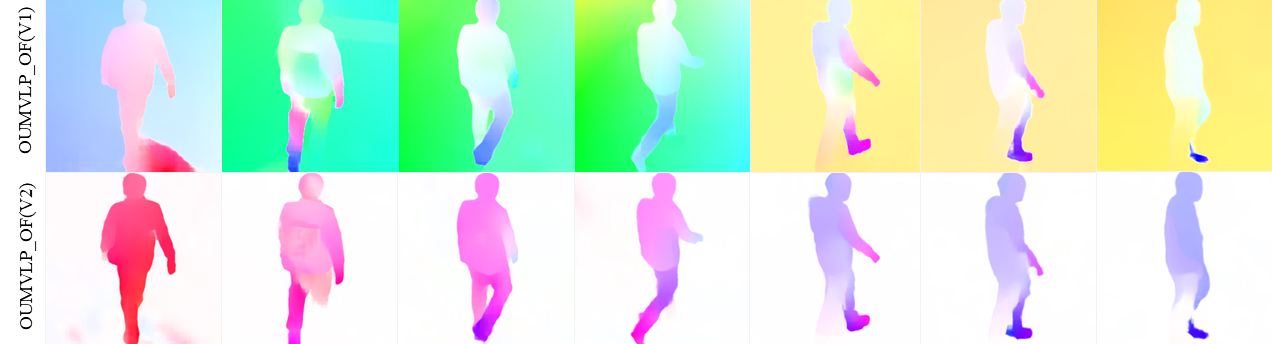
Fig. 1: Samples from the estimated OUMVLP-OF dataset from arbitrary view
We provide two versions of the estimated optical flow maps which described as follows:
OUMVLP-OF (V1)
- This version comprises optical flow maps extracted from RGB sequences that retain raw, noisy backgrounds. The inclusion of background noise is intentional, serving as a form of external data augmentation. By preserving this noise, the dataset encourages models to focus on the intrinsic motion dynamics of gait rather than relying on superficial visual cues or colormap representations. Furthermore, we anticipate that OF-V1 will be particularly beneficial for training larger models, which are more prone to overfitting. The inherent variability introduced by the background noise acts as a natural regularization mechanism, enhancing the model’s generalization capability.
OUMVLP-OF (V2)
- This version consists of optical flow maps with clean, noise-free backgrounds. By leveraging corresponding binary silhouettes, background noise is effectively removed, preserving only the essential gait information. Designed to help models focus solely on gait dynamics, OF-V2 provides a controlled dataset that enhances the analysis of motion patterns, making it particularly useful for extracting fine-grained features.
We will provide optical flow maps compiled from each version as probe and gallary sets, consisting of 2,500 randomly selected subjects for each version.
These probe and gallary sets are intended for evaluation and benchmarking. Participants will use to assess model performance under standardized conditions, ensuring fair and consistent comparisons.
No training samples will be provided, and identity labels have been removed to prevent identity hacking. As a result, participants are free to use any external dataset, such as CASIA-B and GREW (Gait-in-Wild), etc., to generate optical flow maps and train their models.
We will release the data for two phases. For both phases the 2,500 gallary samples will be provided. However, In phase 1, only 20% of the probe set will be released to participants. The remaining 80% will be released in phase 2. To get the passwords to access the files, participants should download, sign, and submit the license aggreemnt to oumvlpof.ch@gmail.com. Once the participants recieved the passwords, they can access the data using the following download links:
License Agreement (IMPORTANT: The Team Leader must sign. If you are student, your supervisor must sign.)
- OUMVLP-OF (V1)
- Gallary set
- Probe set phase 1
- Probe set phase 2
- OVMVLP-OF (V2)
- Gallary set
- Probe set phase 1
- Probe set phase 2
Two Competition Tracks
Track 1: Gait Identification
The goal of this track is to develop models that extract individuals’ identity embeddings from their gait patterns using optical flow maps. The identification scenario works as follows: given a probe optical flow map of an unknown individual, X, and a gallery dataset containing optical flow maps of multiple known individuals, the model should generate an identity embedding for the probe that is closest to the identity embedding of X within the gallery. The models will be evaluated under various walking directions to assess their robustness and generalization capabilities.
Evaluation Protocol for Track 1
The primary metric is the Rank-1 identification rate, which is the probability that the closest match is correct. This metric reflects the one-to-many nature of the identification scenario.
Track 2: Gait Verification
In this track, models similar to those used in the previous track-1 will be applied to the verification scenario. In this setting, both the probe and gallery contain a single gait optical flow map. To determine whether the two maps belong to the same individual, the distance between their identity embeddings is checked.
Evaluations will be conducted under various walking directions to assess robustness and generalization capabilities.
Evaluation Protocol for Track 2
The models will be assessed using the Equal Error Rate (EER), the point at which the False Acceptance Rate (FAR) and False Rejection Rate (FRR) are equal. This metric reflects the one-to-one nature of the identification scenario.
Organizing Committee
- Dr. Allam Shehata, Osaka University, Japan
- Dr. Mohamad Ammar Alsherfawi Aljazaerly, Osaka University, Japan
- Prof. Md Atiqur Rahman Ahad, University of East London, UK
- Prof. Shiqi Yu, Southern University of Science and Technology, China
- Prof. Francisco M. Castro, University of Malaga, Spain
- Prof. Nicol´as Guil, University of Malaga, Spain
- Prof. Manuel J. Marin-Jimenez, University of Cordoba, Spain
- Prof. Yasushi Yagi, Osaka University, Japan
Awards
We are excited to recognize the outstanding achievements of the top three teams in this competition with the following rewards:
- Award Certificates: All members of the winning teams will receive official certificates of achievement.
- IJCB submission Co-Authorship: Up to three members per team (from the 1st, 2nd, and 3rd-place teams) will be invited as co-authors on a competition-related paper to be submitted to the International Joint Conference on Biometrics (IJCB).
This is a unique opportunity to gain academic recognition, contribute to cutting-edge research, and enhance your professional profile.